7 Transformative AI Infrastructure Trends for 2024
Written by Itay Inbar
Decades of technological advancements have converged to ignite a revolution in AI infrastructure.
Larger, faster, and cheaper models boasting several modalities continue to emerge, powered by a new sector of hungry startups vying for a slice of the massive AI-Infrastructure pie.
We expect 2024 to be no different with regards to innovation and increased enterprise and consumer adoption, which will in turn drive much needed advancement. Here are our seven trends we believe to be shaping the future of this landscape.
1. Compute Limitations to Drive Innovation in Underlying Infrastructure
Increasingly larger and more compute intensive models, alongside persistent bottlenecks in compute accessibility and performance, are driving innovation across the infrastructure spectrum for both training and inference workloads.
As a result, we are witnessing efforts to accelerate AI from nearly every direction – dedicated hardware accelerators for AI, faster storage and networking at the data-center level, software-based model and compute optimizers, and tools for enhanced resource utilization.
For example, Greenfield Portfolio company DustPhotonics is enabling next-gen optical communications in the data center to support the ever-increasing speed requirements brought upon by AI.
2. Models Will Increasingly Become Multimodal
The progression towards multimodal models (ones that can analyze a variety of different input types, such as text, images, videos, and scientific instrument data) are gaining momentum, with companies like OpenAI’s GPT and DALL-E integrations, and Google’s Gemini leading the charge through impressive advancements.
This shift is opening doors to a myriad of new applications, broadening the horizon of AI's capabilities at the application level for both enterprises and consumers.
3. Both Closed and Open-Source Models will Thrive
The landscape of LLMs is set for a diverse ecosystem where both open and closed-source models will experience significant growth in popularity.
Major players like OpenAI in partnership with Microsoft, Anthropic with AWS, and Google’s Gemini are poised to consolidate their market share, with OpenAI maintaining a leading position.
Enterprises are expected to prefer the reliability and support of closed-source models for internal applications, while we expect product development to lean towards open-source models, motivated by the need to circumvent higher costs.
4. RAG will Continue to Emerge as the Leading Architecture Blueprint for Customized LLMs
The Retrieval-Augmented Generation (RAG) model is solidifying its position as the primary architecture blueprint for customizing LLMs to one’s specific needs, a trend expected to continue due to its advantages in ease and cost of setup, data freshness, and minimized hallucinations. As model providers look to broaden their offering, vector databases, which are integral to RAG architecture, are anticipated to integrate seamlessly into LLM APIs, evolving into a RAG-as-a-service model, gaining in popularity for those looking for ease and simplicity.
5. Increased Investment in Data Platforms as an Enabler of AI
Enterprises are ramping up investments in data platforms, recognizing them as essential enablers of AI deployment. This trend reflects a growing emphasis on establishing organized, centralized data repositories to facilitate efficient AI search capabilities and foster trust in underlying data.
Specifically, we expect to see increased spend on centralized data lakes/warehouses, and a greater emphasis placed on data quality, observability, and governance.
Innovations like those of Greenfield portfolio company VAST Data, with its novel AI data platform, architected from the ground up to power AI and GPU-accelerated applications, will continue to move the space forward.
6. Enabling the Enterprise to Deploy AI at Scale
While enterprises are eagerly looking to deploy AI within their organizations, enabling AI at enterprise-scale requires addressing key challenges such as access control, user management, monitoring, privacy, security, and regulatory compliance, jointly named by Gartner as AI TriSM (Trust, Risk, and Security Management).
As large organizations integrate AI, governing its usage becomes a growing concern. We expect initial investments in this space to focus on RBAC, privacy, and model monitoring. However, proactive measures against genuine cybersecurity threats are expected to gain priority only in subsequent years, marking a gradual shift towards comprehensive AI security strategies.
7. Bridging Software Development and AI with Specialized Tools
Traditionally, AI and machine learning have been realms dominated by specialized data science and AI engineering teams, with a strong emphasis on statistical analysis, model training, and data processing. However, with the advent of accessible API-based LLMs, traditional software engineers are increasingly called upon to integrate these capabilities into a broader range of applications and platforms.
This shift necessitates a new generation of development tools enabling software engineers to leverage AI functionalities efficiently without requiring the deep specialized knowledge that has historically been the purview of data scientists.
--
As an active investor in data infrastructure and AI applications, we look forward to continuing to engage with up-and-coming market leaders in the space and taking part in enabling its growth. If you’re building in this space, please don’t hesitate to reach out.
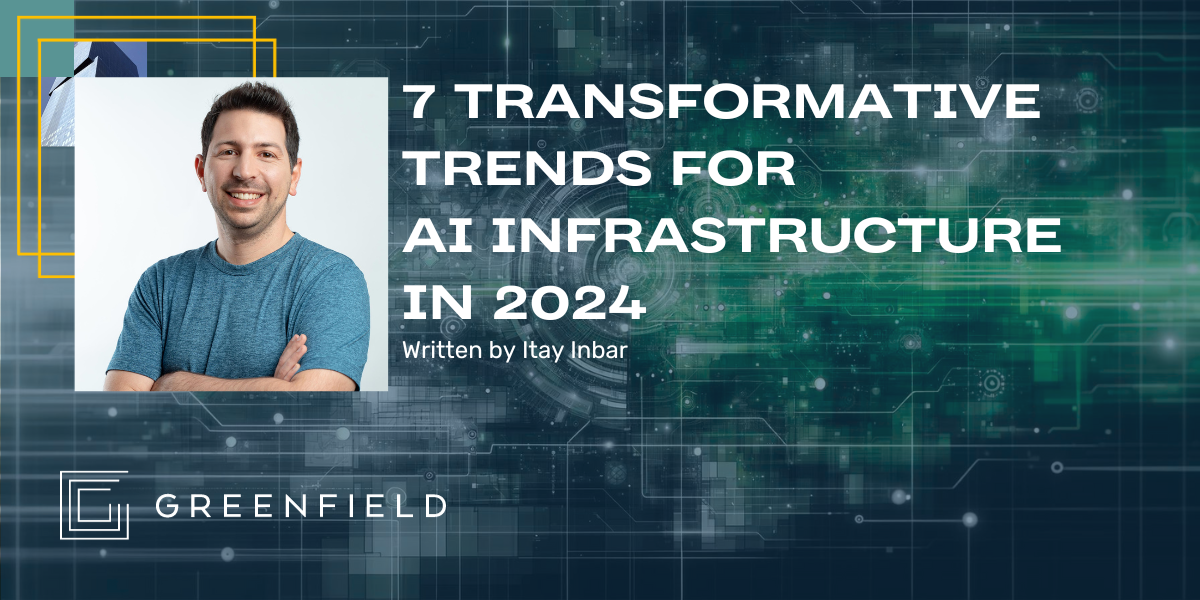